Full report
The science of prognostics has been going through a rapid and fruitful development in the past decades, with various forecasting methods, procedures and approaches flooding the economic world. It is estimated that there are more than 100 prediction methods, and sometimes the diversity makes it difficult to choose the one that would do the trick. In our new research we try and compare a few of the most popular techniques, and see if they are in fact suitable for forecasting currency exchange rates.
Results
The 5-day window SMA model gives the worst results in our analysis. The model captures the general direction of the series and the main peaks and troughs, but is not sensitive to small changes. We can thus say that the result is too smoothed. Which is hardly surprising since the forecast is just the average of the previous values. The exponential smoothing, on the other hand, gives much better results. The EMA curve not only moves in the right direction, but also shows even the small motions of the price. In this case a lot depends on the choice of the constant α. We use α=0.8, therefore putting the largest weight on the last observation. Visually, however, it is obvious that the best results come from ARIMA(1,1,1), DLM, and ANN(3-2-1) - their predictions practically coincide (see Figure 4).
All forecasting curves are lagged behind the real values. On of the reasons for this is the strong autocorrelation in the series, but the main cause of the forecast delay is the huge weight of the previous observation in the models. All mentioned techniques increasingly depend on the last value of the time series, with SMA and ANN being the only exceptions. SMA5 puts equal weights on all five last observations, while ANN fits weights values for the three previous data points. As this forecasting bias seems to be rather considerable, it is natural to wonder whether it is altogether worth it to take trouble over the models. Thus we compare our results to the ones we would obtain by simply assuming the present value of the prediction to be equal to the observation in the previous time moment.
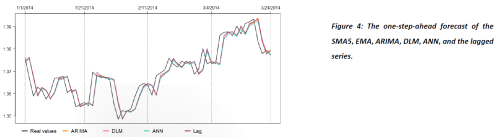
Conclusion
We have studied five forecasting models with different construction techniques and levels of difficulty. In total, all received results followed the general motion of the real series, some showing slightly more precision than others. Notably, the forecast given by the classical ARIMA model was even better than the results of more complex DLM and ANN. As ARIMA is also easier and faster to employ, the superior results make it unquestionably the best forecasting method in our study. However, SMA and EMA models are also very simple in both understanding and realisation, with SMA giving the most smooth curves, but EMA being more dynamic. So if the high precision of the forecast is not the main target of analysis, both these methods can also be used.
It is noteworthy, however, that all the models give lagged, or delayed, predictions, and do not offer much superiority over a simple lagged series. Such outcome is dictated by the nature of time series – it is non-stationary and strongly autocorrelated. Therefore, in cases when pointwise and indefinably accurate predictions would suffice, it might be more sensible to save time and effort, and use the lagged series.
The science of prognostics has been going through a rapid and fruitful development in the past decades, with various forecasting methods, procedures and approaches flooding the economic world. It is estimated that there are more than 100 prediction methods, and sometimes the diversity makes it difficult to choose the one that would do the trick. In our new research we try and compare a few of the most popular techniques, and see if they are in fact suitable for forecasting currency exchange rates.
Results
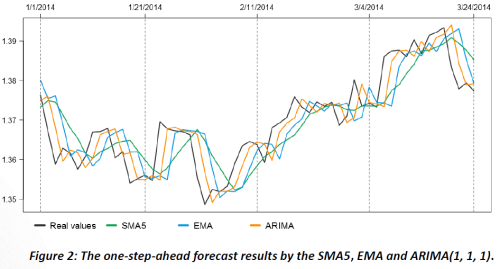
The 5-day window SMA model gives the worst results in our analysis. The model captures the general direction of the series and the main peaks and troughs, but is not sensitive to small changes. We can thus say that the result is too smoothed. Which is hardly surprising since the forecast is just the average of the previous values. The exponential smoothing, on the other hand, gives much better results. The EMA curve not only moves in the right direction, but also shows even the small motions of the price. In this case a lot depends on the choice of the constant α. We use α=0.8, therefore putting the largest weight on the last observation. Visually, however, it is obvious that the best results come from ARIMA(1,1,1), DLM, and ANN(3-2-1) - their predictions practically coincide (see Figure 4).
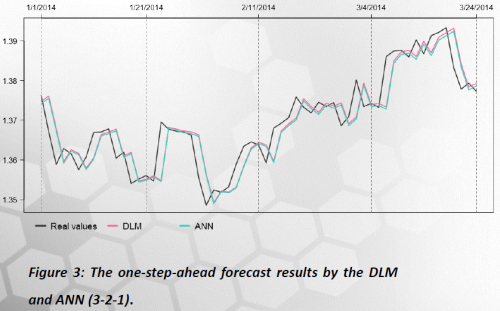
All forecasting curves are lagged behind the real values. On of the reasons for this is the strong autocorrelation in the series, but the main cause of the forecast delay is the huge weight of the previous observation in the models. All mentioned techniques increasingly depend on the last value of the time series, with SMA and ANN being the only exceptions. SMA5 puts equal weights on all five last observations, while ANN fits weights values for the three previous data points. As this forecasting bias seems to be rather considerable, it is natural to wonder whether it is altogether worth it to take trouble over the models. Thus we compare our results to the ones we would obtain by simply assuming the present value of the prediction to be equal to the observation in the previous time moment.
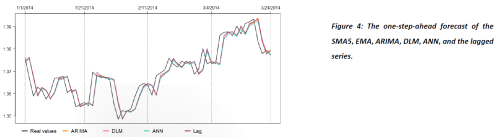
Conclusion
We have studied five forecasting models with different construction techniques and levels of difficulty. In total, all received results followed the general motion of the real series, some showing slightly more precision than others. Notably, the forecast given by the classical ARIMA model was even better than the results of more complex DLM and ANN. As ARIMA is also easier and faster to employ, the superior results make it unquestionably the best forecasting method in our study. However, SMA and EMA models are also very simple in both understanding and realisation, with SMA giving the most smooth curves, but EMA being more dynamic. So if the high precision of the forecast is not the main target of analysis, both these methods can also be used.
It is noteworthy, however, that all the models give lagged, or delayed, predictions, and do not offer much superiority over a simple lagged series. Such outcome is dictated by the nature of time series – it is non-stationary and strongly autocorrelated. Therefore, in cases when pointwise and indefinably accurate predictions would suffice, it might be more sensible to save time and effort, and use the lagged series.